2.1 Comparison between Statistician and Data Scientist
Statistics as a scientific area can be traced back to 1749, and statistician as a career has been around for hundreds of years with well-established theory and application. Data scientist becomes an attractive career for only a few years, along with the fact that data size and variety beyond the traditional statistician’s toolbox and the fast-growing of computation power. Statistician and data scientist have a lot in common, but there are also significant differences, as highlighted in figure 2.1.
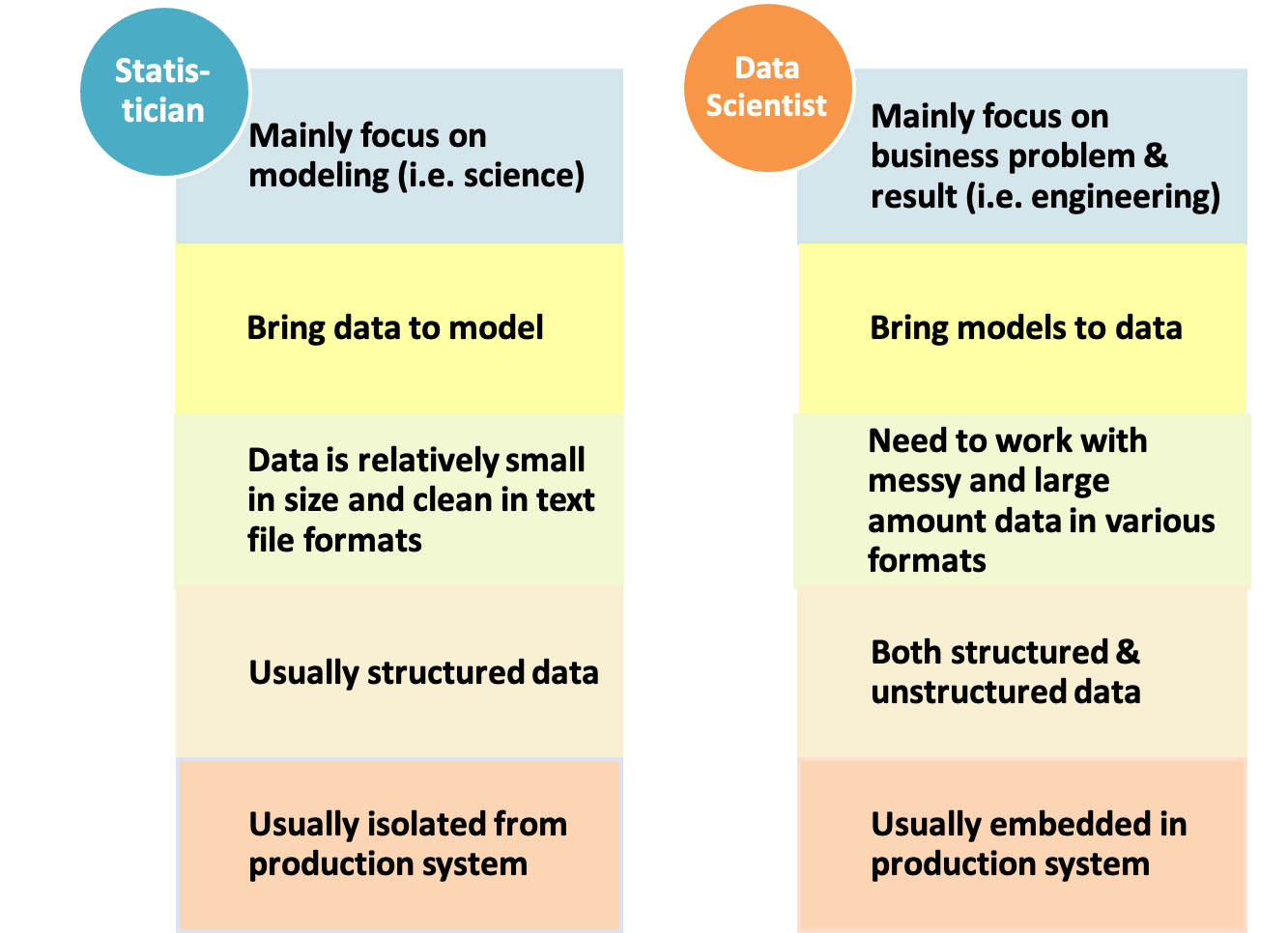
FIGURE 2.1: Comparison of statistician and data scientist
Both statisticians and data scientists work closely with data. For typical traditional statisticians, the data set is usually well-formatted text files with numbers (i.e., numerical variables) and labels (i.e., categorical variables). The data’s size is typically small enough to be loaded in a PC’s memory or be saved in a PC’s hard disk. Comparing to statisticians, data scientists need to deal with more varieties of data:
- well-formatted data stored in a database system with a size much larger than a PC’s memory or hard-disk;
- a huge amount of verbatim text, voice, image, and video;
- real-time streaming data and other types of records.
One unique power of statistics is to make statistical inferences based on a small set of data. Statisticians, especially in academia, usually spend most of their time developing models and don’t need to put too much effort into data cleaning. However, data becomes relatively abundant recently, and modeling is (often small) part of the overall effort. Due to open source communities’ active development, fitting standard models are not too far away from button-pushing. Data scientists in industry instead spend a lot of time preprocessing and wrangling the data before feeding them to the model.
Unlike statisticians, data scientists often focus on delivering actionable results and sometimes need to deploy the model to the production system. The data available for model training can be too large to be processed in a single computer. From the entire problem-solving cycle, statisticians are usually not well integrated with the production system where data is obtained in real-time, while data scientists are more embedded in the production system and closer to the data generation procedures. In summary, statisticians focus more on modeling and usually bring data to models, while data scientists focus more on data and usually bring models to data.